Prediction of Salt in Soil by PLS Regression Using Hyperspectral Laboratory Data
Tejas U. Padghan1 , Ratnadeep R. Deshmukh2 , Jaypalsing N. Katye3 , Anita G. Khandizod4 , Pooja V. Janse5
Section:Research Paper, Product Type: Journal Paper
Volume-7 ,
Issue-2 , Page no. 172-175, Feb-2019
CrossRef-DOI: https://doi.org/10.26438/ijcse/v7i2.172175
Online published on Feb 28, 2019
Copyright © Tejas U. Padghan, Ratnadeep R. Deshmukh, Jaypalsing N. Katye, Anita G. Khandizod, Pooja V. Janse . This is an open access article distributed under the Creative Commons Attribution License, which permits unrestricted use, distribution, and reproduction in any medium, provided the original work is properly cited.
View this paper at Google Scholar | DPI Digital Library
How to Cite this Paper
- IEEE Citation
- MLA Citation
- APA Citation
- BibTex Citation
- RIS Citation
IEEE Citation
IEEE Style Citation: Tejas U. Padghan, Ratnadeep R. Deshmukh, Jaypalsing N. Katye, Anita G. Khandizod, Pooja V. Janse, “Prediction of Salt in Soil by PLS Regression Using Hyperspectral Laboratory Data,” International Journal of Computer Sciences and Engineering, Vol.7, Issue.2, pp.172-175, 2019.
MLA Citation
MLA Style Citation: Tejas U. Padghan, Ratnadeep R. Deshmukh, Jaypalsing N. Katye, Anita G. Khandizod, Pooja V. Janse "Prediction of Salt in Soil by PLS Regression Using Hyperspectral Laboratory Data." International Journal of Computer Sciences and Engineering 7.2 (2019): 172-175.
APA Citation
APA Style Citation: Tejas U. Padghan, Ratnadeep R. Deshmukh, Jaypalsing N. Katye, Anita G. Khandizod, Pooja V. Janse, (2019). Prediction of Salt in Soil by PLS Regression Using Hyperspectral Laboratory Data. International Journal of Computer Sciences and Engineering, 7(2), 172-175.
BibTex Citation
BibTex Style Citation:
@article{Padghan_2019,
author = {Tejas U. Padghan, Ratnadeep R. Deshmukh, Jaypalsing N. Katye, Anita G. Khandizod, Pooja V. Janse},
title = {Prediction of Salt in Soil by PLS Regression Using Hyperspectral Laboratory Data},
journal = {International Journal of Computer Sciences and Engineering},
issue_date = {2 2019},
volume = {7},
Issue = {2},
month = {2},
year = {2019},
issn = {2347-2693},
pages = {172-175},
url = {https://www.ijcseonline.org/full_paper_view.php?paper_id=3639},
doi = {https://doi.org/10.26438/ijcse/v7i2.172175}
publisher = {IJCSE, Indore, INDIA},
}
RIS Citation
RIS Style Citation:
TY - JOUR
DO = {https://doi.org/10.26438/ijcse/v7i2.172175}
UR - https://www.ijcseonline.org/full_paper_view.php?paper_id=3639
TI - Prediction of Salt in Soil by PLS Regression Using Hyperspectral Laboratory Data
T2 - International Journal of Computer Sciences and Engineering
AU - Tejas U. Padghan, Ratnadeep R. Deshmukh, Jaypalsing N. Katye, Anita G. Khandizod, Pooja V. Janse
PY - 2019
DA - 2019/02/28
PB - IJCSE, Indore, INDIA
SP - 172-175
IS - 2
VL - 7
SN - 2347-2693
ER -
![]() |
![]() |
![]() |
640 | 512 downloads | 254 downloads |
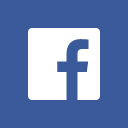
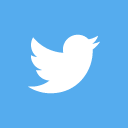
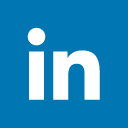
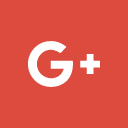
Abstract
the intent of this paper is prediction of the salt content in agriculture soil. Soil salinity is a process which affects the quality of soil and reduces the agriculture production. The soil salt content adversely affects the soil physical property including soil water content. The Visible and Near-Infrared Reflectance Spectroscopy provides improved estimation of soil salinity as fast approach to the characterization of soil salt content with spectral resolution of 350-2500 nm. The Partial Least Square Regression Method (PLSR) is frequently used to determine Soil Salt Content (SSC) obtains from the spectral data. The Result shows that the 550nm, 850 nm, 1430nm, 1918nm, 2052nm wavelength which are sensitive to salt content and model based on Partial Least Square Regression PLSR can only make approximate predictions for First Derivative RMSE (Root Mean Square Error) = 0.0282-0.0365, R2 (Coefficient of Determination) = 0.9313-0.9051 and for Continuum Remove RMSE (Root Mean Square Error) = 0.0280-0.0386, R2 (Coefficient of Determination) = 0.9313-0.8939.
Key-Words / Index Term
Spectral Data, Visible-NIR, Soil Salt Content (SSC), Partial Least Square Regression (PLSR).
References
[1] Jun Wang, Zhoujing Li, XuebinQin, XiuchengYang, ZhonglingGao, Qiming Qin, “Hyperspectral predicting model of soil salinity using partial least square regression’’ © IEEE 2014.
[2] Ashwini D. Padmbhai, R. R. Deshmukh “Hyperspectral Analysis of Soil Total nitrogen,” International Journal of Advance Resesrch in Computer Science Vol 8, No. 7, July – August 2017.
[3] DongZhang, TashpolatTiyip, JianliDing, FeiZhang, “Quantitative Estimating Salt Content of Saline Soil Using Laboratory Hyperspectral Data” Hindawi Publishing 14Sept 2016.
[4] YaLiu, XianzhangPan, ChangkunWang “predicting of soil salinity with VIS-NIR spectra,” journal.pone.0140688 Oct 15, 2015.
[5] Shibin Liu,Yi Li and chansheng, “Spectral estimation of soil salt content,” soilsci April 2, 2013.
[6] Farifteh J., F. Van der Meer, C. Atzberg, and E. Carranza, “Quantitative analysis of salt-affected soil reflectance spectra A comparison of two adaptive methods (PLSR),” Remote Sensing Environment, vol. 110, pp. 59-78, 2007.
[7] Yogling Weng., P. Gong, and Z. Zhu, “Reflectance spectroscopy for the assessment of soil salt content in soils of the Yellow River Delta of China,” International Journal Of Remote Sensing, vol. 29, pp. 5511-5531, Feb2, 2008.
[8] Metternicht G. and J. Zinck, “Remote sensing of soil salinity potentials and constraints,” Remote Sensing Of Environment, vol. 85, pp.Jan 1, 2003.
[9] S. Nawar, H. Buddenbaum, J. Hill, “Estimation of soil salinity using three quantitative methods based on Vis-NIR reflectance spectroscopy a case study from Egypt,” Arabian Journal of Geosciences, vol. 8, no. 7, pp. 5127–5140, 2015.
[10] Amal Allbed. “ Soil Salinity Mapping and Monitoring,” Scip journal oct 2013.
[11] Harris J.K. “Spectral identification and Quantification of Salt”, Remote sensing 2016.
[12] Taha Gorji, Aysegul Tanik, “Soil Salinity Prediction”, Elsevier, Volume 15, 2015.
[13] Hela Tiriki. “Modeling of soil salinity Using Spectral Analysis,” Geoscience 2015.
[14] Dnyaneshwar D. Karale R.R.Deshmukh, “Estimation of Soil Organic Carbon Content using Visible Near Infrared Reflectance Spectroscopy,” International Journal of Advance Reserch In Computer Science,vol 8, No. 5, May-June 2017.
[15] J. Peng, W. J. “Predicting total dissolved salts and soluble ion concentrations in agricultural soils using visible near-infrared spectrometers,” Biosystems Engineering, 2016.
[16] F. Zhang, T. Tiyip, J. Ding et al., “Studies on the reflectance spectral features of saline soil along the middle reaches of TarimRiver: a case study in Xinjiang Region, China,” Environmental Earth Sciences, vol. 69, no. 8, pp. 2743–2761, 2015.
[17] E. K. D. Abd et al., “Detecting salinity hazards within a semiarid context by means of combining soil and remote-sensing data,” Geoderma 134, 217–230 2006.
[18] L. Lei et al.“Study on the soil salinization monitoring based on measured hyperspectral and HSI data,” Spectral Analysis. 34, 1948–1983, 2014.
[19] S. Ayetiguli et al., “Estimating soil salinity in Pingluo county of China using QuickBird data and soil reflectance spectra,” Int. J. Appl. Earth Obs. Geoinform. 26, 156–175 (2014).
[20] N. M. Khan and Y. Sato, “Monitoring hydro-salinity status and its impact in irrigated semiarid areas using IRS-1B LISS-II data,” Asian J. Geoinform 3, 63–73 2001.
[21] Madhurama Sethi “Salinity appraisal using IRS image in upper krishna Irrigation project karnatka”, International Journals of Remote Sensing, Vol. 27, No. 14, 2917–2926; 20 July 2006.
[22] Rachel Lugaci “Studying Vegetation Salinity from the Field View to a Satellite-Based Perspective”, Remote Sensing, 2017.
[23] D. Wang, C. Wilson and M. C. Shannon, “Interpretation of Salinity and Irrigation Effects on Soybean Canopy Re- flectance in Visible and Near-Infrared Spectrum Domain,” International Journals of Remote Sensing, Vol. 23, No. 5, pp. 811-824.2002.
[24] S. Teggi et al. “SPOT 5 Imagery for Soil Salinity As-sessment in Iraq,” Remote sensing, Vol. 8538,pp. 85380-85380, 2012.
[25] F. Iqbal, “Detection of Salt Affected Soil in Rice-Wheat Area Using Satellite Image,”African Journals of Agriculture Reserch,Vol. 6, No.21, pp.4973-4982, 2011.